How To Select The Right Master Data Management Framework For Your Organization
Master Data Management (MDM) is crucial for organizations that want to ensure data accuracy and consistency across all their systems and applications. However, with so many MDM frameworks available in the market, selecting the right one for your organization’s specific needs can be overwhelming.
This article will provide you with a comprehensive guide to choosing the right MDM framework for your organization.
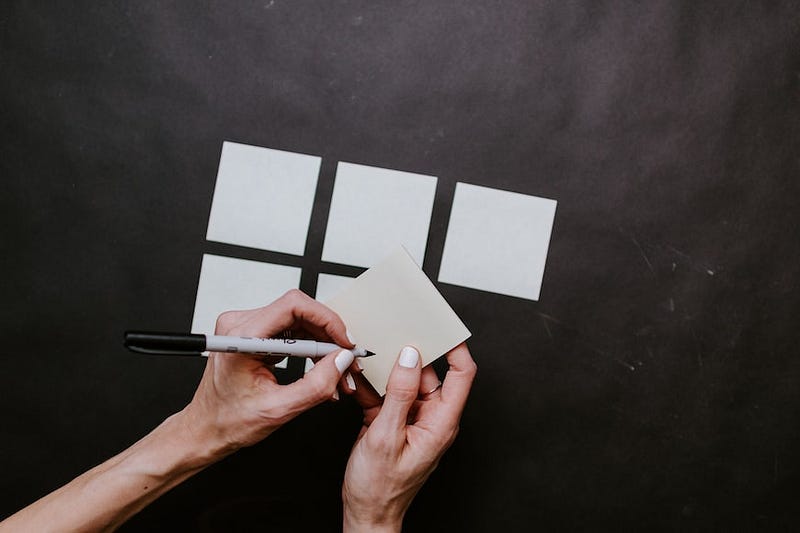
Master data management is critical in today’s data-driven business landscape: it enables organizations to keep their data accurate, consistent, and complete across all systems and departments. This provides them with a foundation for healthy decision-making. At its core, master data management is about maintaining a single source of truth for all stakeholders to reference.
However, it is not a one-size-fits-all solution.
The way in which organizations approach master data management depends on a variety of factors, including the size and complexity of their data, what they aim to do with it, and their organizational structure. To help you choose the best master data management framework for your needs, we’ve summarized three of the most prevalent models along with their advantages and disadvantages.
1. Multi-domain Master Data Management
In industry speak, a data “domain” is simply a type of data. For example, customer data, product data, and location data are all domains.
If you have critical data spread across multiple domains and need to maintain a high level of control and governance over that data, your best bet is probably a multi-domain, or cross-domain, master data management framework. This will allow you to manage multiple types of domain data within a single platform.
There are a few different ways to implement multi-domain solutions, but the most common approach is to use a centralized repository. This repository acts as a single source of truth for all of your organization’s critical data. Data from each domain is loaded into the repository and is then synchronized across all domains.
Advantages
The major advantage of this approach is that it gives you a complete view of your organization’s master data, with integrations happening in real-time. For instance, a multi-domain system can help you manage relationships between data, such as customer-to-product or product-to-location. This can be valuable for understanding cross-sell and upsell opportunities or for managing inventory levels.
In addition, keeping a centralized view of all master data makes it easier to identify errors and inconsistencies.
Disadvantages
Multi-domain master data management does pose some challenges. First and foremost, it can be very complex and often requires administration by a dedicated cross-functional team, which may or may not be realistic depending on your organization’s culture and capacity. Multi-domain master data management solutions can also be expensive for small businesses.
Young companies with a limited amount of data to govern may opt to start with smaller-scale solutions, then graduate to a multi-domain framework as their needs evolve.
2. Hub-and-Spoke Master Data Management
Hub-and-spoke frameworks can be useful for organizations that have critical data in one domain (a “hub”) with some secondary data in other domains (“spokes”).
For example, an organization might have a customer master data hub that is used to manage core customer data. This hub would then be connected to multiple spoke systems, each containing secondary data about customers, such as a billing system, a CRM system, and an e-commerce system.
These relationships are managed by data sharing and synchronization rules that are defined in the hub.
Advantages
Hub-and-spoke frameworks offer some centralization of data management while retaining flexibility. The spoke systems can be connected and disconnected from the hub as needed without affecting the overall structure of the framework. This can be helpful if an organization needs to add or remove a secondary system.
Another advantage is that each system only needs to send updates to the hub when data changes, which can decrease the amount of duplicate data across systems. In turn, this can reduce the time and effort needed to manage data.
Disadvantages
The disadvantage of a hub-and-spoke framework is that it can be more complex than other models, especially for fast-growing organizations that have a large number of spoke systems. This is because each system needs to be connected to the hub, which can require additional development work.
Additionally, if the hub goes down, then the entire system will be affected. This can be a major issue for organizations that rely on their data being available at all times.
A further downside of hub-and-spoke master data management is that you don’t get a complete view of your organization’s data. For example, customer and product data will not appear in the same view, meaning you may miss out on the chance to analyze cross-domain relationships.
3. Single-domain Master Data Management
Many of the master data management solutions on the market are based on governing a single entity, such as product data. This is also known as a point solution. A single-domain master data management framework manages only one master data domain and is not concerned with other types of data in the company.
Advantages
This framework may suffice for companies that only need to manage a single type of master data, that do not require that data be shared with other applications or business units, and that do not intend to expand their master data management capacity in the future. Single-domain solutions typically involve simple architecture, making them quicker and easier to set up than other models.
Free Book: Practical Guide to Implementing Entity Resolution
Interested in implementing an in-house record matching solution with your own development team without using any outside vendors or tools?
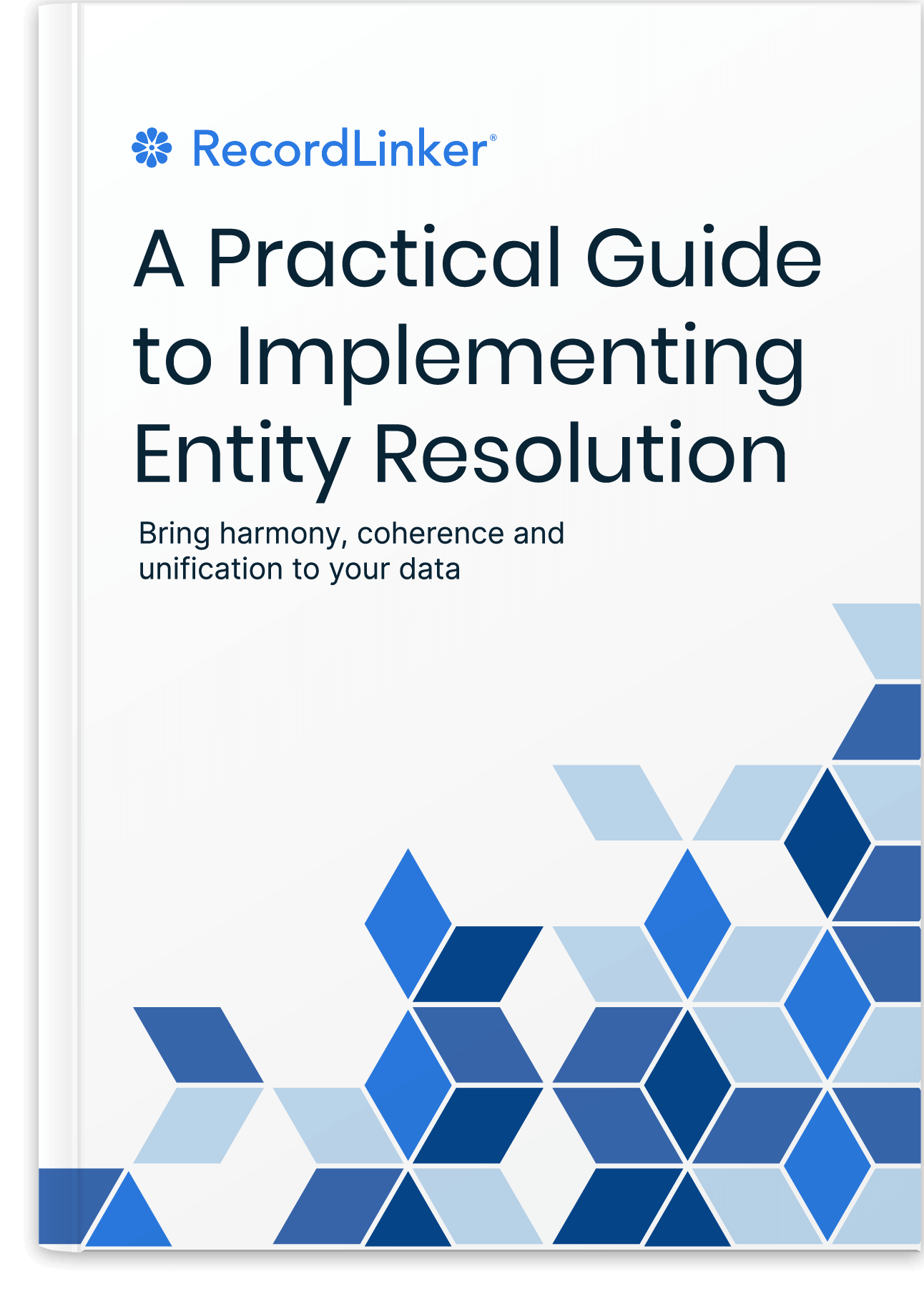
They can be a good first step for small businesses that want to improve their data management practices but are not yet ready to adopt a multi-domain solution.
Disadvantages
The main drawback of the single-domain framework is the lack of integration. Critical data remains isolated from other data management processes and technologies in the organization. This can lead to data silos and a lack of visibility on data relationships. Additionally, a lack of centralized oversight can make implementing data security and quality control measures more challenging.
In Summary
Choosing the best master data management framework for your organization requires thinking carefully about the types and complexity of critical data you handle, what you intend to do with that data, and the capacity of your organization to support the demands of each model.
No matter which framework you choose, make sure you’ve covered best practices for master data management implementation, including clearly defining what qualifies as master data and introducing measures to keep data consistent, secure, and up to date.
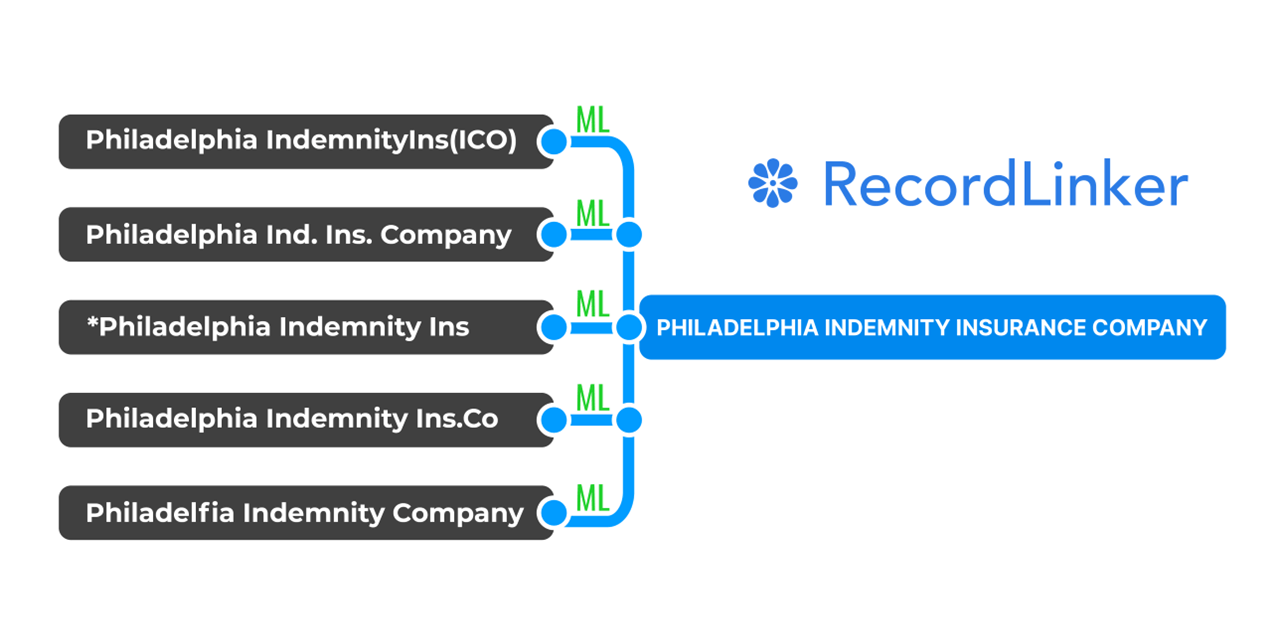
Interested in improving the quality of your data but don’t have the time or resources to create a master data management program from the ground up? RecordLinker is here to help. Our data integration and management platform can quickly connect disparate data sources, identify and deduplicate records, and keep your data clean and up-to-date.