Master Data Management Implementation: Best Practices
Are you looking for an efficient way to manage your most critical data? Master Data Management implementation is the answer. With its goal of creating a single, authoritative source for all key data elements, it can help organizations make sound decisions and reduce costs.
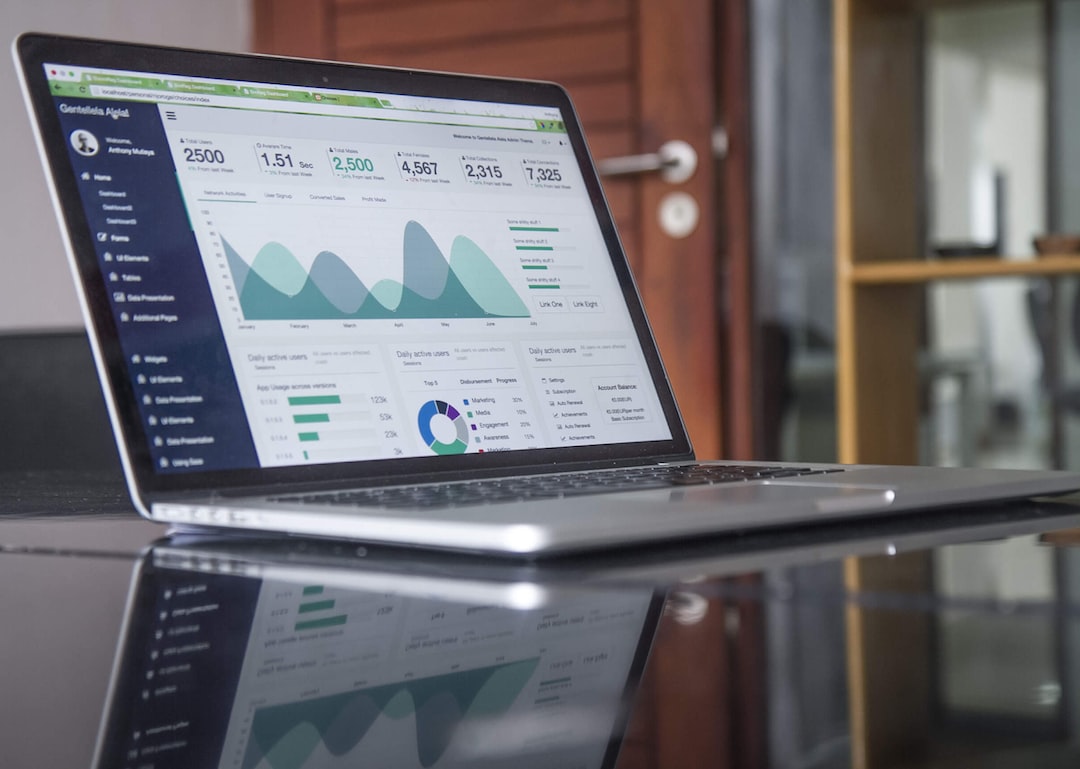
For any organization to work efficiently, make sound decisions, and reduce costs, it needs accurate and timely information about its customers, products, suppliers, and the other key entities in its ecosystem. Unfortunately, data is too often managed in a haphazard manner with little or no governance in place.
A 2021 survey showed that while 84% of global business leaders saw a need for greater data-driven insights, 55% had misgivings about the reliability of their own data. This can lead to inconsistency and errors, which can have a cascading effect on downstream processes and decision-making.
The best way to avoid getting off track is through master data management implementation, a comprehensive approach to managing an organization’s most critical data. Its goal is to create a single, authoritative source for all key data elements. It’s never too early or late to start thinking about how you can improve the way you manage master data. To help you find the right approach, here are five best practices.
1. Define What Master Data Is and Isn’t
In order to establish a focused and efficient master data management system, we need to define what should qualify as “master data.” In a nutshell, think of it as any data that is shared by multiple departments and used to drive key business decisions. A good rule of thumb is to ask yourself whether a certain piece of data is used in more than one business process. If the answer is yes, then it’s likely master data.
Common examples of master data include product details, customer and employee profiles, and supplier information. On the other hand, “transactional data,” such as invoices, sales records, and customer service interactions, is relevant only for specific, fairly closed processes. It also tends to be dynamic and short-lived.
Differentiating between these types of data is an important step in master data management implementation. It enables you to scope the project and focus your efforts where they will have the most impact.
2. Assemble a Cross-Functional Team
When it comes to master data management, no one has all the answers. Assemble a center of excellence from different areas of your organization to get a well-rounded perspective on your data. This team may include representatives from IT, data governance, business operations, finance, and line-of-business managers.
Together, they will be responsible for the master data management implementation, including establishing levels of access and creating rules for data monitoring, governance, and use. The team will need resources and authority to make decisions that affect the whole organization. They will also need to connect regularly with other teams to ensure that everyone uses the same data standards.
3. Cleanse Your Data
Cleansing data simply means identifying and correcting inaccuracies or inconsistencies in your record-keeping. This is a crucial step in master data management implementation because it ensures that all downstream processes are working with accurate, complete data. For instance, you may encounter differences in the way your data has been formatted between systems or across departments.
You may have some systems that store dates as DD/MM/YYYY and others that store them as MM/DD/YYYY.
These inaccuracies can come from any number of sources, including manual entry errors, system glitches, or outdated information. You’ll need to establish a list of standard formats and apply it consistently across all of your data. Cleansing your data manually is often error-prone, tedious, and extremely time-consuming.
Consider using a machine-learning-based data harmonization tool to link records thoroughly and efficiently across multiple systems.
Free Book: Practical Guide to Implementing Record Linkage
Interested in implementing an in-house record linkage solution with your own development team without using any outside vendors or tools?
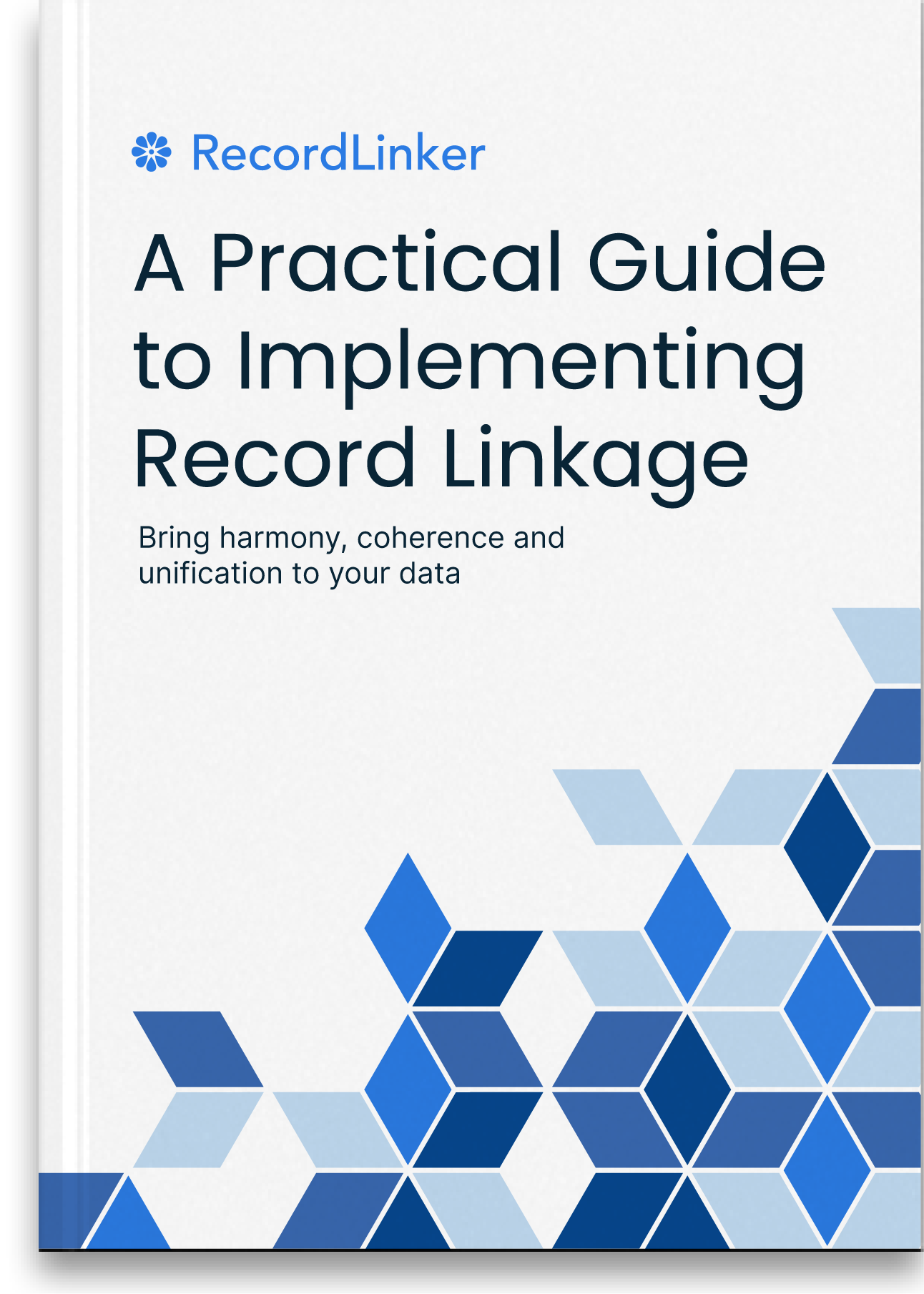
4. Enrich Your Data
Enriching data involves adding new data that was not previously present or augmenting existing data with new information. When deciding if and where data enrichment is needed, think through the business processes that will be supported by the master data. Where could adding additional context or detail make the biggest impact?
For example, you may be interested in enriching your customer profiles with social media, demographic data, firmographics, or other sources. This helps to create a complete view of the customer, which can be valuable for decision-making across multiple departments.
Data enrichment can be performed manually through research or surveys, but you can also find software to help with the process of applying new information to a data set.
5. Introduce Security Measures
Data security is a critical aspect of master data management implementation. If your organization handles sensitive information, make sure to implement proper security protocols early on in the process.
Your first priority should be classifying your data. You need to understand what information is most sensitive and take the appropriate steps to protect it. This may include encrypting certain data sets or using access control measures to restrict who can see and edit certain information.
Next, you need to develop a system for managing data security breaches. No matter how well you secure your data, there’s always a risk of theft or unauthorized access. Be prepared for worst-case scenarios by having a plan in place for how you will respond to a breach. This should include steps for immediately securing any exposed data and launching an investigation to determine how the breach occurred.
Finally, don’t forget to train your employees on data security best practices. They should know how to handle sensitive information and what to do in the event of a security breach. Regular training can help reduce the risk of human error, which is often the cause of data leaks.
In Summary
Master data management implementation takes time, commitment, and a forward-looking mentality. It also takes a village. By clearly defining the scope of your project, engaging a cross-departmental team, and taking steps to cleanse, enrich, and secure your data, you’ll create a strong foundation for decision-making that inspires confidence, improves collaboration, and reduces stress.
Interested in improving the quality of your data but don’t have the time or resources to create a master data management program from the ground up? RecordLinker is here to help. Our data integration and management platform can quickly connect your disparate data sources, identify and deduplicate records, and keep your data clean and up-to-date.