Data Quality Dimensions: The Key To Useful Data
Data turns into a liability when it’s collected and kept as an afterthought – without direction and care.
To benefit from data, organizations need to maintain it and ensure its quality. Data quality dimensions are the structured way of looking at different properties of data assets. Understanding them enables business to systemically approach increasing data quality and work on bridging gaps where poor quality comes from.
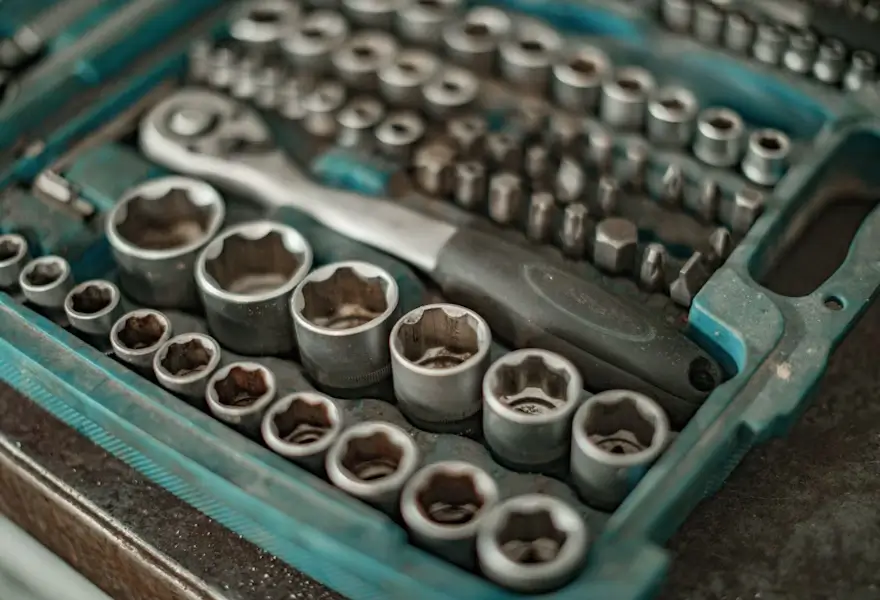
Data is the foundation of informed decision-making and operations. From small startups to multinational corporations – businesses rely on data to gain insights, drive growth, and stay competitive. The value of data hinges not just on its quantity but also on its quality.
What Is Data Quality?
Data quality refers to the degree to which data meets the requirements of its intended use. In other words, it’s about how reliable, accurate, and relevant your data is for making informed decisions and driving business processes. Think of data quality as the measure of how trustworthy and valuable your data is in supporting your organization’s goals and objectives.
How Do You Improve Data Quality?
Data quality is about having the right data in the right condition. When data is of poor quality—riddled with errors, inconsistencies, or missing pieces—it becomes unreliable and can lead to faulty analyses, misguided decisions, and operational inefficiencies. Therefore, ensuring high data quality is essential for maximizing the value of your data assets and maintaining a competitive edge in today’s data-driven landscape.
This is where the concept of data quality dimensions comes into play.
Improving data quality involves systematically addressing various aspects or dimensions of data. By focusing on these properties, organizations can assess the quality of their data assets, identify areas for improvement, and implement targeted strategies to enhance data quality over time.
What Are The Main Data Quality Dimensions?
Data quality dimensions provide a structured framework for evaluating and improving the reliability, accuracy, and relevance of data. By addressing them, organizations can enhance the trustworthiness of their data assets and ensure that they are aligned with business objectives. Moreover, maintaining high-quality data becomes more and more essential for mitigating risks and ensuring regulatory compliance.
There are 6 data quality dimensions that are the most widespread – while not necessarily considered principal. These include:
- accuracy
- completeness
- consistency
- uniqueness
- timeliness
- validity
By prioritizing data quality, businesses can improve collaboration and alignment across departments, ensuring that everyone is working towards common data quality goals. Ultimately, paying attention to data quality dimensions is not just about mitigating risks; it’s about unlocking the full potential of data to drive growth, innovation, and competitive advantage.
On top of these benefits, addressing data quality enables growth of data-driven culture within organizations. When decision-makers have confidence in the quality of the data they are using, they are more likely to rely on data-driven insights to guide their strategic initiatives. This not only leads to more informed decision-making but also supports innovation and agility.
Let’s take a look at the individual dimensions in detail.
1. Data Accuracy
Accuracy is one of the fundamental dimensions of data quality. It refers to the degree to which data correctly represents the real-world objects or events it is intended to describe.
Inaccurate data can lead to misguided decisions and operational inefficiencies. For instance, imagine a retail company relying on inaccurate sales figures to plan inventory. The consequences could include overstocking of certain items and shortages of others, resulting in lost revenue and dissatisfied customers. To improve accuracy, businesses can implement simple measures such as data validation checks to identify and correct errors before they impact decision-making processes.
Tips for Achieving Accurate Data
- Implement data validation checks at the point of entry to catch and correct errors immediately.
- Utilize data profiling tools to identify potential inaccuracies and inconsistencies.
- Conduct regular data quality assessments to identify areas for improvement.
- Train staff on the importance of accurate data entry and provide ongoing support.
- Establish clear data quality metrics and targets to measure accuracy over time.
2. Data Completeness
Completeness is another crucial dimension of data quality. It refers to the extent to which all required data elements are present within a dataset. When thinking about completeness, think about blank or null values.
Incomplete data can hinder analysis and lead to incomplete or inaccurate insights. For example, a marketing campaign based on incomplete customer profiles may fail to target the right audience effectively. To ensure completeness, businesses can employ practical strategies such as setting mandatory fields in data entry forms to capture essential information consistently.
Tips for Achieving Complete Data:
- Set mandatory fields in data entry forms to capture essential information consistently.
- Regularly review data collection processes to identify gaps in completeness.
- Implement automated alerts for missing data elements.
- Conduct periodic data quality assessments to ensure completeness.
- Utilize data profiling tools to identify incomplete datasets.
3. Data Consistency
Consistency is vital for maintaining data quality. It involves ensuring uniformity and coherence across different datasets or within the same dataset.
Inconsistent data can lead to confusion and misinterpretation, undermining the reliability of analysis and decision-making. Common sources of inconsistency include variations in data formats and naming conventions. To promote consistency, businesses can adopt basic techniques such as standardizing data formats and enforcing naming conventions consistently across systems and processes.
Tips for Achieving Consistent Data
- Standardize data formats and naming conventions across systems and processes.
- Implement data governance policies to enforce consistency.
- Establish data quality rules to identify and resolve inconsistencies.
- Train staff on the importance of maintaining consistent data.
- Conduct regular data quality audits to monitor consistency.
4. Data Uniqueness
Data uniqueness is a critical dimension of data quality that ensures each data element within a dataset is distinct and lacks redundancy. It involves the identification and removal of duplicate records or entries, thereby preventing ambiguity and confusion in data analysis and decision-making processes.
By maintaining data uniqueness, organizations can enhance the reliability and accuracy of their data, facilitating more informed insights and strategic initiatives. Strategies for ensuring data uniqueness include implementing unique identifiers, establishing data governance policies, conducting regular data cleansing, and utilizing data quality tools to automate duplicate detection and resolution. Ultimately, prioritizing data uniqueness enables businesses to maximize the value of data assets and drive better outcomes.
Tips for Achieving Uniqueness in Data
- Implement unique identifiers to ensure each data element is distinct.
- Establish data governance policies for managing duplicate records.
- Conduct regular data cleansing to remove redundant entries.
- Utilize data quality tools to automate duplicate detection and resolution.
- Train staff on best practices for maintaining data uniqueness.
5. Data Timeliness
Timeliness is a critical dimension of data quality, especially in fast-paced business environments. It is about timely delivery of data, or the delay between the moment when something happens and gets captured as data into the data set.
Timeliness is about regular data collection in respect of business needs. There could be data you need in real time (think about stock market), and there could still be data that does not require day-to-day attention.
While timeliness may result with data becoming outdated, it’s not a measure of how close to reality data is i.e. timeliness is not interested whether data is outdated or reflects reality well. These areas belong to currency – however it’s common to hear people treating timeliness in this broader sense.
Tips for Efficient Work with Timeliness
- Implement regular data updates to ensure information is current.
- Utilize automated data feeds to capture real-time data.
- Conduct regular audits to monitor data freshness.
- Implement alerts for outdated or stale data.
6. Data Validity
Validity is essential for ensuring the integrity and reliability of data. It refers to the extent to which data conforms to defined rules or constraints, ensuring its logical and structural soundness. Invalid data can compromise the accuracy and trustworthiness of analyses and reports. For instance, a healthcare provider relying on invalid patient records may encounter difficulties in providing accurate diagnoses and treatments. To ensure validity, businesses can implement user-friendly methods such as implementing data integrity constraints and validation rules.
Tips for Achieving Valid Data
- Implement data validation rules at the point of entry to ensure data conforms to defined standards.
- Utilize data quality tools to perform automated checks for data validity and flag any discrepancies.
- Establish data governance policies to enforce data validity standards and ensure compliance.
- Conduct regular audits of data sources and processes to identify potential validity issues.
- Provide ongoing training and support to staff to promote adherence to data validity standards and best practices.
Other Data Quality Dimensions
Naturally, you will most likely come upon other data quality dimensions or properties of data. Here’s a quick summary of dimensions in use:
- relevance – how useful data is for what it is intended, without irrelevant information contaminating it
- precision – how rounded, detailed, or granular data is
- reliability – how trustworthy data is, how well it can lead to reliable results and conclusions
- accessibility – how easy to access and understand data is
- currency – whether data captured in a data set matches the current real-world context i.e. captured data could describe a state that does not exist any longer
- conformity – whether attributes belonging to the same set are using consistent formats and data types
Problems with Data Quality Dimensions
A word of caution – data quality dimensions absolutely can do harm. Remember that by adopting these you decide for a certain world view. When approached and enforced in a zealous way, they can hinder effective work with data. When data quality dimensions end up overdone, they introduce excessive complexity and “artistry” in data management processes.
For example, strict requirements for data accuracy and validity may result in extensive data validation procedures and data cleansing efforts, can slow down data processing and analysis. Similarly, rigid standards for data completeness may lead to delays in data collection and integration, hindering timely decision-making.
The pursuit of perfection in data quality can create unrealistic expectations and standards that are difficult to achieve in practice. This can result in frustration among data professionals and stakeholders, leading to resistance to data-driven initiatives and undermining the overall effectiveness of data management efforts.
While data quality dimensions are essential for ensuring the reliability and usability of data, organizations must strike a balance between ensuring data quality and maintaining agility and efficiency in data management processes.
Suggested Reading About Data Quality and Management
Welcome to the world of conscious approach to data. It’s a long journey, it’s not easy – but it’s worth it! Check our recommended reading list for resources that will help you establish good data practices in your organization, changing how you manage and use data for better.
- Data Cleansing and Normalization for Better Insights
- Taking Care of Data with Data Stewardship
- Matching Data in Excel: Why You May Regret This
- Matching Names of Companies, Vendors, Suppliers, And More!
- Planning Your Data Migration: Best Practices
- Creating a Canonical Record Set For Efficient Data Management
- 5 Key Components of Master Data Management
Driving Better Outcomes Through Data Quality Dimensions
Data quality dimensions play a critical role in ensuring the reliability, usefulness, and trustworthiness of data for decision-making and operations.
By addressing each dimension systematically, businesses can enhance data quality and unlock the full potential of their data assets. Prioritizing data quality efforts is essential for driving better outcomes, fostering innovation, and maintaining a competitive edge and market agility.
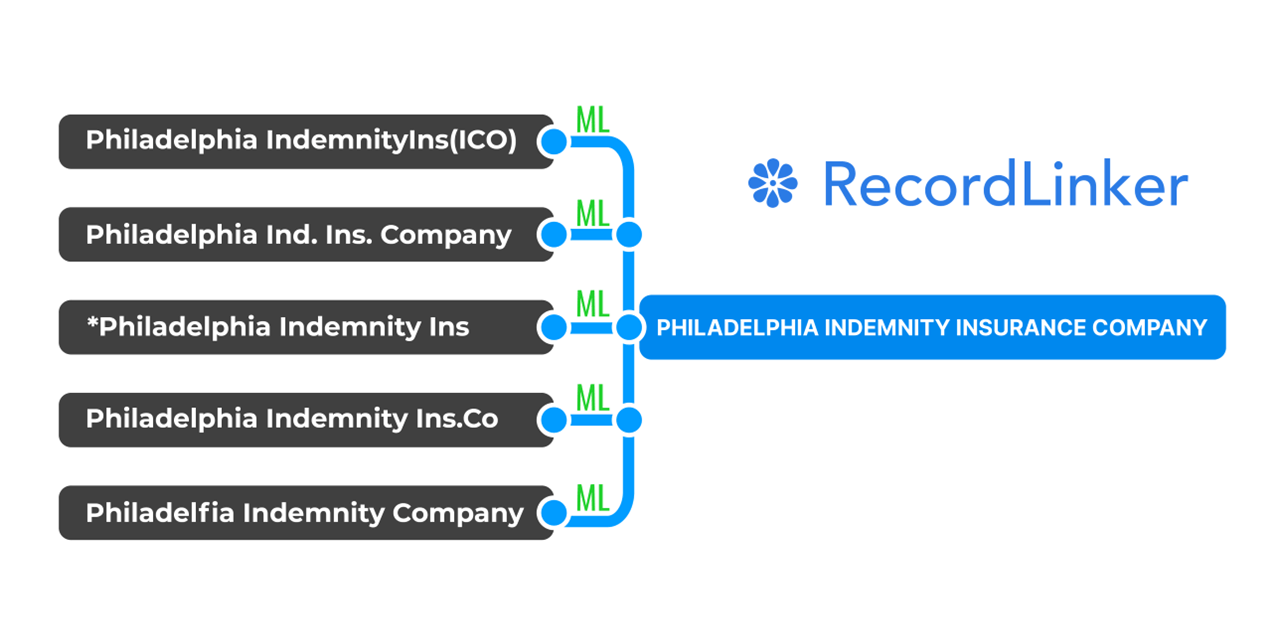
RecordLinker uses Machine Learning to normalize records across your data systems!
Interested in improving the quality of your data? You can improve your capacity for keeping your data accurate, consistent, and linked throughout disparate systems. It’s a good way to take the brunt of tedious work off your data stewards, admins, and data conversion specialists in a super scalable and user-friendly way.
RecordLinker can help create your master data management program without the need to build it completely from ground-up. Our data matching and management platform can quickly connect your disparate data sources, identify and deduplicate records, and keep your data clean and up-to-date.
To learn more about how RecordLinker can help you improve the quality of your data, request a free demo!